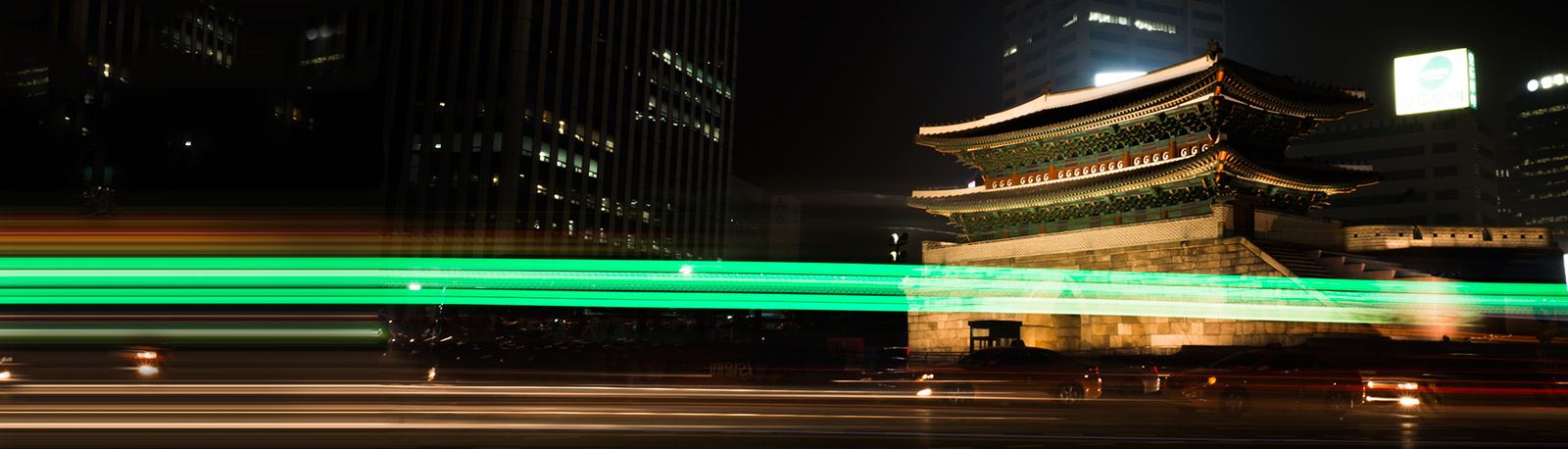
APRIL 18-21, 2017
News
Sponsors
Previous Events
Channels
Logo
You can download the logo here.
|
DrugPathSeeker: Interactive UI for Exploring Drug-ADR Relation via PathwaysAbstractBiological interpretation and understanding of machine learning based predictive models are highly desirable in healthcare analytics. Predicting Adverse Drug Reactions (ADRs) is extremely important for safe and precision medicine. There are various machine learning based approaches to predict adverse reactions for drugs. These models, though effective, lack biological interpretation and are treated as black-boxes. We propose DrugPathSeeker, a novel interactive user interface that integrates the machine learning model, database query API, statistical analysis, and visualization for exploring and understanding of the association between drugs and ADRs. The proposed UI can take a query drug, and provide a visual interface designed to support exploration of the predictions from the machine learning model for further understanding and interpretation. DrugPathSeeker uses a machine learning model, Small Molecular Risk Profiler, to make ADR predictions for a given drug. The visualization uses Sankey type flow diagrams for highlighting the relation between the drugs and ADRs. The main goal of Drug-PathSeeker is to mine the gene-pathways from public databases and analyze them in a visual manner to generate a biological hypothesis. DrugPathSeekerテ「竄ャ邃「s effectiveness is demonstrated with two use cases: mechanisms of action for carbamazepine-induced dystonia, and fluorometholone-induced diabetes mellitus |