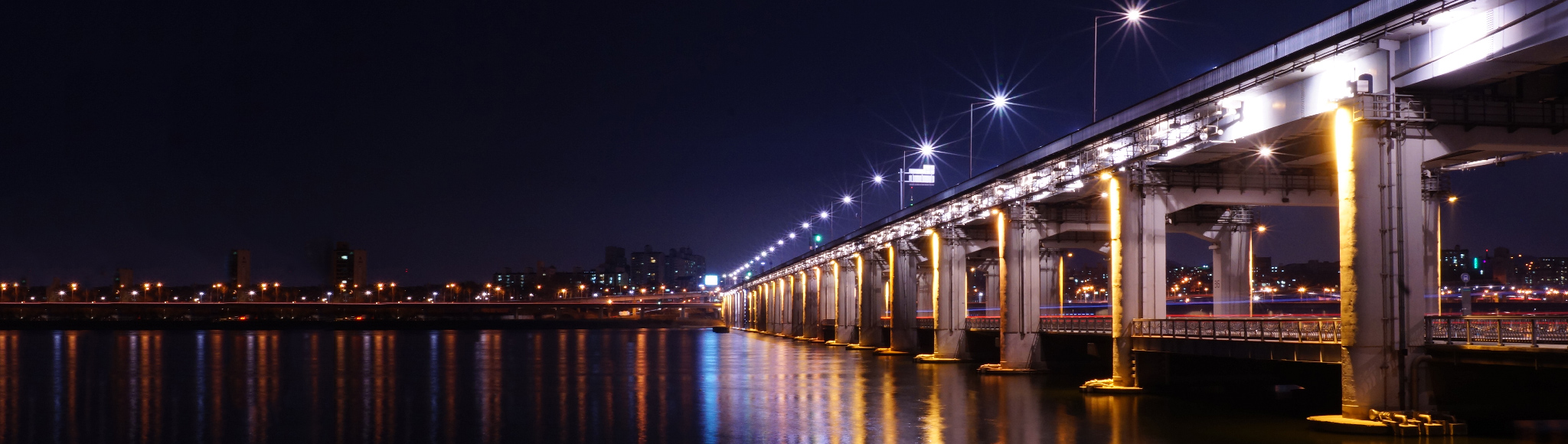
APRIL 18-21, 2017
News
Sponsors
Previous Events
Channels
Logo
You can download the logo here.
|
Aeonium: Visual Analytics to Support Collaborative Qualitative CodingAbstractQualitative coding offers the potential to obtain deep insights into social media, but the technique can be inconsistent and hard to scale. Researchers using qualitative coding impose structure on unstructured data through 窶彡odes窶 that represent categories for analysis. Our visual analytics interface, Aeonium, supports human insight in collaborative coding through visual overviews of codes assigned by multiple researchers and distributions of important keywords and codes. The underlying machine learning model highlights ambiguity and inconsistency. Our goal was not to reduce qualitative coding to a machine-solvable problem, but rather to bolster human understanding gained from coding and reinterpreting the data collaboratively. We conducted an experimental study with 39 participants who coded tweets using our interface. In addition to increased understanding of the topic, participants reported that Aeonium窶冱 collaborative coding functionality helped them reflect on their own interpretations. Feedback from participants demonstrates that visual analytics can help facilitate rich qualitative analysis and suggests design implications for future exploration. |