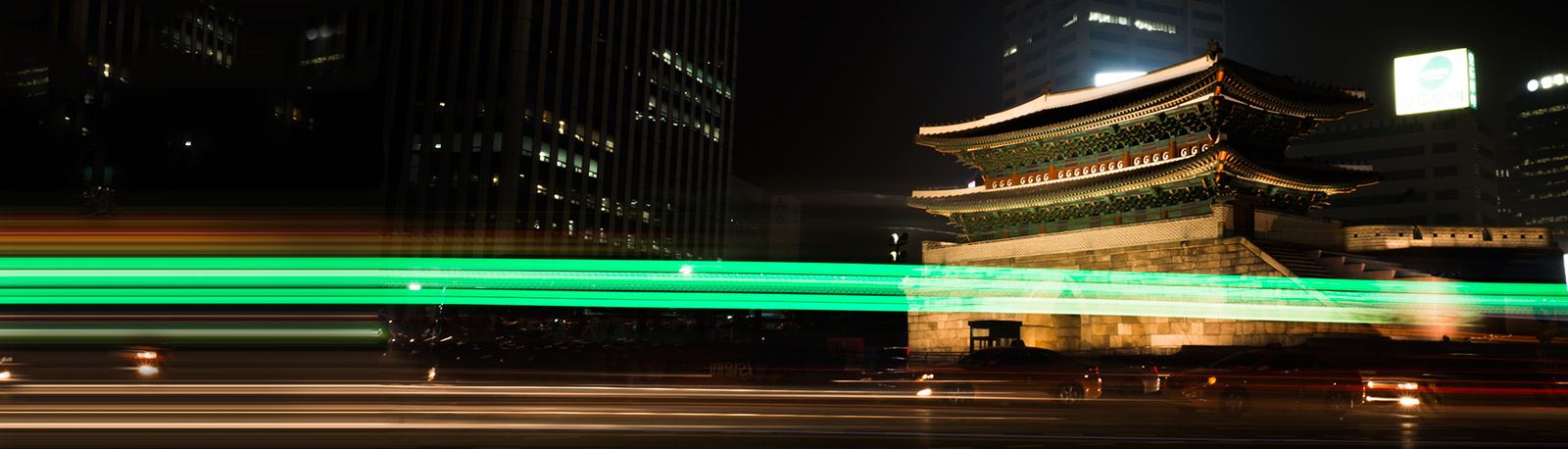
APRIL 18-21, 2017
News
Sponsors
Previous Events
Channels
Logo
You can download the logo here.
|
Statistical Visualization and Analysis of Large Data Using a Value-based Spatial DistributionAbstractThe size of large-scale scientific datasets created from simulations and computed on modern supercomputers continues to grow at a fast pace. A daunting challenge is to analyze and visualize these intractable datasets on commodity hardware. A recent and promising area of research is to replace the dataset with a distribution based proxy representation that summarizes scalar information into a much reduced memory footprint. Proposed representations subdivide the dataset into local blocks, where each block holds important statistical information, such as a histogram. A key drawback is that a distribution representing the scalar values in a block lacks spatial information. This manifests itself as large errors in visualization algorithms. We present a novel statistically-based representation by augmenting the block-wise distribution based representation with location information, called a value-based spatial distribution. Information from both spatial and scalar spaces are combined using Bayes' rule to accurately estimate the data value at a given spatial location. The representation is compact using the Gaussian Mixture Model. We show that our approach is able to preserve important features in the data and alleviate uncertainty. |