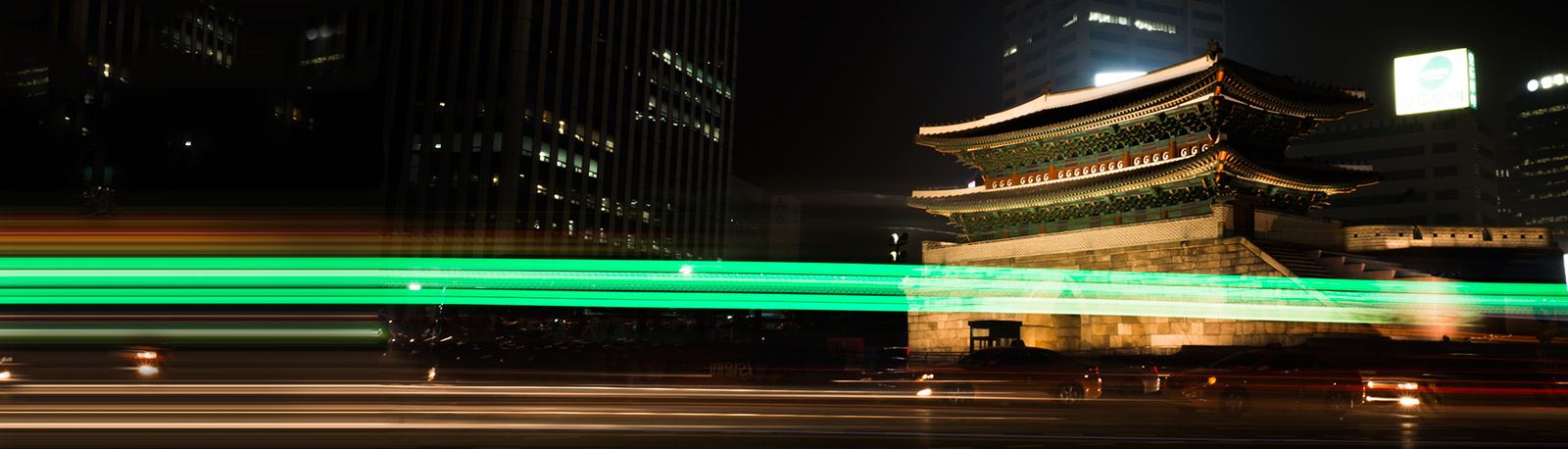
APRIL 18-21, 2017
News
Sponsors
Previous Events
Channels
Logo
You can download the logo here.
|
Range Likelihood Tree: A Compact and Effective Representation for Visual Exploration of Uncertain Data SetsAbstractUncertain data visualization plays a fundamental role in many applications such as weather forecast and analysis of fluid flows. Exploring scalar uncertain data modeled as probability distribution fields is a challenging task because the underlying features are often more complex, and the data associated with each grid point are high dimensional. In this work, we present a compact and effective representation, called range likelihood tree, to summarize and explore probability distribution fields. The key idea is to decompose and summarize each complex probability distribution over a few representative subranges by cumulative probabilities, and allow users to consider the roles that different subranges play in understanding the probability distributions. In our method, the value domain is first partitioned into subranges, then the distribution at each grid point is transformed according to the cumulative probabilities of the point's distribution in those subranges. Organizing the subranges into a hierarchical structure based on how these cumulative probabilities are spatially distributed in the grid points, the new range likelihood tree representation allows effective classification and identification of features through user query and exploration. We present an exploration framework with multiple interactive views to explore probability distribution fields, and provide guidelines for visual exploration using our framework. We demonstrate the effectiveness and usefulness of our approach in exploratory analysis using several representative uncertain data sets. |