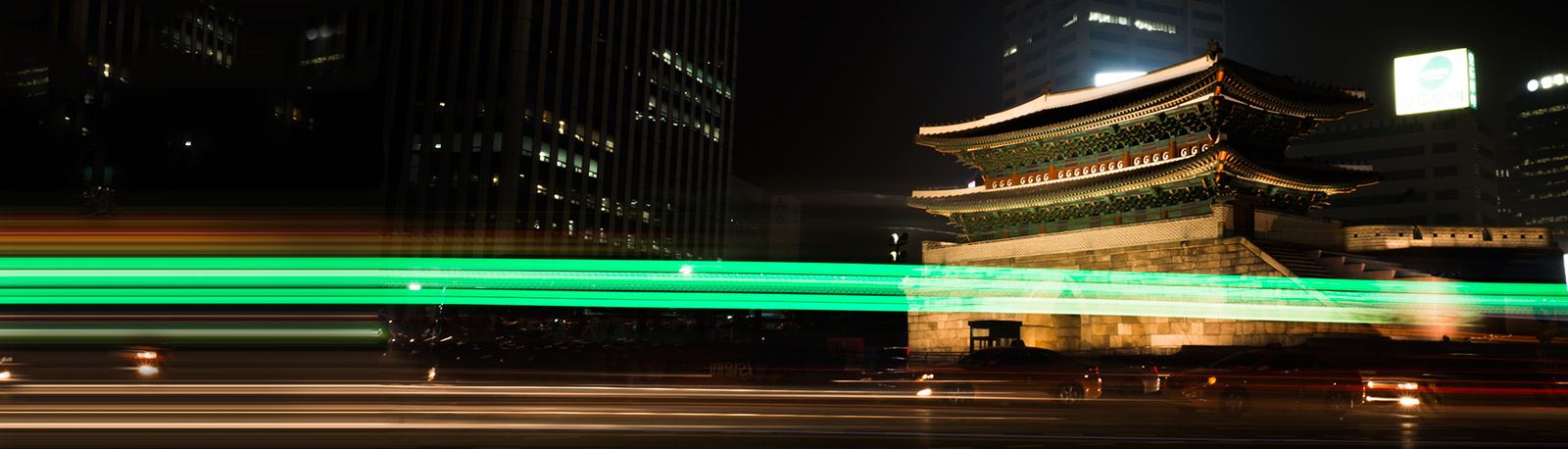
APRIL 18-21, 2017
News
Sponsors
Previous Events
Channels
Logo
You can download the logo here.
|
Multivariate Volumetric Data Analysis and Visualization through Bottom-Up Subspace ExplorationAbstractMultivariate volumetric datasets are often encountered in results generated by scientific simulations. Compared to univariate datasets, analysis and visualization of multivariate datasets are much more challenging due to the complex relationships among the variables. As an effective way to visualize and analyze multivariate datasets, volume rendering has been frequently used, although designing good multivariate transfer functions is still non-trivial. In this paper, we present an interactive workflow to allow users to design multivariate transfer functions. To handle large scale datasets, in the preprocessing stage we reduce the number of data points through data binning and aggregation, and then a new set of data points with a much smaller size are generated. The relationship between all pairs of variables is presented in a matrix juxtaposition view, where users can navigate through the different subspaces. An entropy based method is used to help users to choose which subspace to explore. We proposed two weights: scatter weight and size weight that are associated with each projected point in those different subspaces. Based on those two weights, data point filter and kernel density estimation operations are employed to assist users to discover interesting features. For each user-selected feature, a Gaussian function is constructed and updated incrementally. Finally, all those selected features are visualized through multivariate volume rendering to reveal the structure of data. With our system, users can interactively explore different subspaces and specify multivariate transfer functions in an effective way. We demonstrate the effectiveness of our system with several multivariate volumetric datasets. |