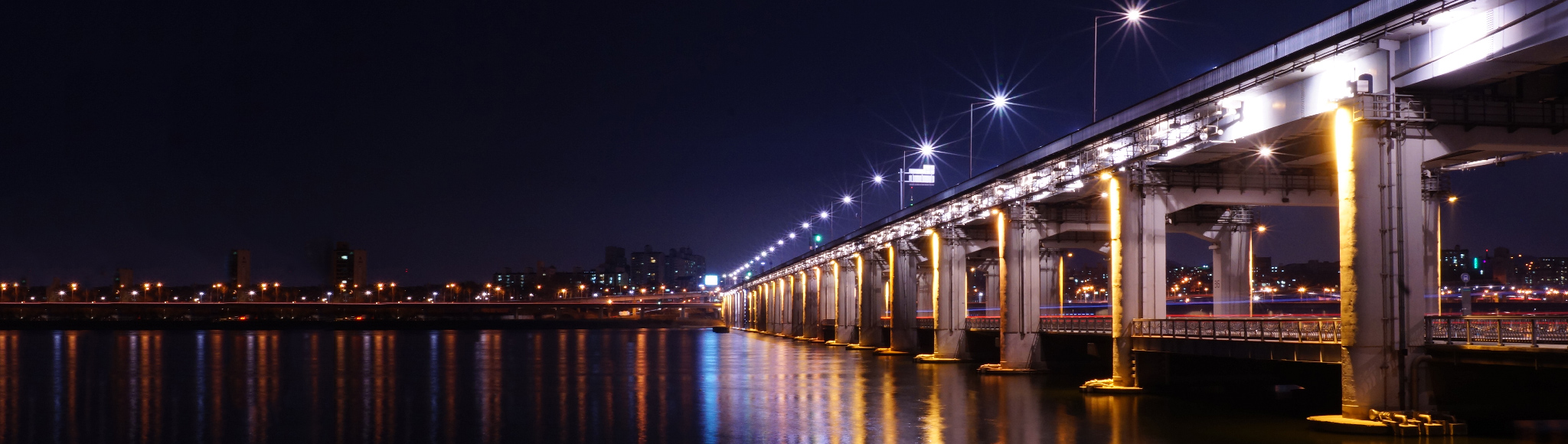
APRIL 18-21, 2017
News
Sponsors
Previous Events
Channels
Logo
You can download the logo here.
|
Efficient Distribution based Feature Search in Multi-field DatasetsAbstractLocal distribution search is used in query-driven visualization for \ identifying salient features. Due to the high computational and \ storage costs, local distribution search in multi-field datasets is challenging. In this paper, we introduce two high performance, memory \ efficient algorithms for searching for local distributions that are \ characterized by marginal and joint features in multi-field datasets. \ They leverage bitmap indexing and local voting to efficiently extract \ regions that match a target distribution, by first approximating \ search results and refining to generate the final result. The first \ algorithm, merged-bin-comparison (MBC), reduces the computation \ of histogram dissimilarity measures by clustering bins. The second \ algorithm, sampled-active voxels (SAV), adopts stratified sampling \ to reduce the workload for searching local distributions with large \ spatial neighborhoods. The efficiency and efficacy of our algorithms \ are demonstrated in multiple experiments. |